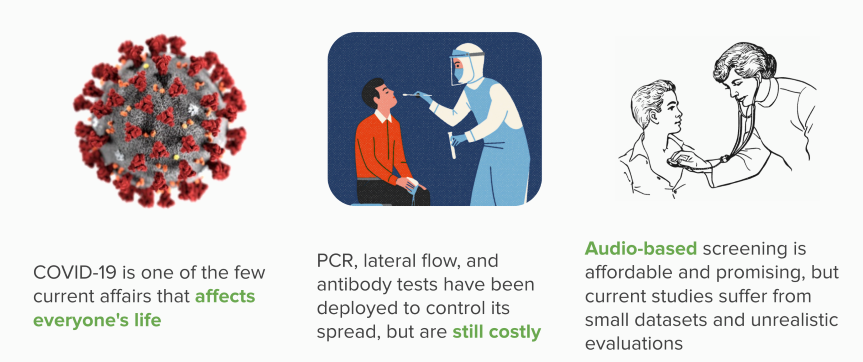
Submitted by Rachel Gardner on Fri, 19/08/2022 - 16:26
As the UK licences a new Covid-19 vaccine ahead of this autumn’s booster campaign, researchers here have unveiled another tool that could help control the spread of the virus.
They have successfully demonstrated a remote tracking technology that can monitor the progression of Covid-19 illness in a patient over time and accurately predict their recovery. They say that this could point the way forward to easy and affordable ways to track this disease and indeed other respiratory illnesses.
This work follows on from the development by the research team of the Covid-19 Sounds app in April 2020 in response to the pandemic.
Since it was set up, over 43,000 participants – both Covid-positive and negative – have used the app to record themselves coughing, breathing, and speaking. These recordings have been used to train machine learning algorithms to diagnose whether people are indeed suffering from Covid-19.
The initial results from the research showed that the deep learning models had a high success rate in detecting Covid-19. And now the researchers have gone further. In their latest work they describe how, that in addition to taking a snapshot of a patient’s health, they were able to use these recordings to successfully track how the illness progressed in patients over time and to predict their recovery from it.
To the best of our knowledge, this is the first work exploring longitudinal audio dynamics for COVID-19 disease progression.
Dr Ting Dang
Their work Exploring Longitudinal Cough, Breath, and Voice Data for COVID-19 Progression Prediction via Sequential Deep Learning: Model Development and Validation was recently published in the Journal of Medical Internet Research, the leading journal for digital medicine and health.
First author Ting Dang (pictured right), a Senior Research Associate here, says: "To the best of our knowledge, this is the first work exploring longitudinal audio dynamics for COVID-19 disease progression prediction."
And the research team says it shows that the app could be a flexible, affordable, and timely tool for COVID-19 tracking over time, showing how the disease progresses and when patients recover from it.
While Covid-19 is an illness that affects everyone, PCR, lateral flow and antibody tests are expensive. The researchers argue that audio-based screening like this – i.e. screening that uses body sounds such as coughing and breathing to diagnose illness – is very promising and could be much more affordable.
The researchers selected for analysis 212 individuals who supplied their breathing, cough, and voice recordings, along with their Covid test results, for periods of time ranging from 5 to 385 days.
They then developed and validated a sequential deep learning-enabled method to detect COVID-19 disease progression.
They wanted to find out if, from analysing particular audio features of these recordings, their model could accurately distinguish between Covid-positive and -negative participants over time, and accurately track how the disease was progressing in those who were Covid-positive.
They were able to verify the predictions of disease progression by comparing the way their model predicted the trajectory of the illness with the actual test results supplied by the participants.
The researchers report that their system performed strongly in distinguishing between COVID-19 positive and negative audio sample. And when it came to predicting the progression of the disease, "the predictions displayed high consistency with the longitudinal test results, with a high correlation of 0.86…"
Its success, they say, suggests that this system has "potential in home monitoring during post-peak and post-pandemic time."
And they think it has wider implications than that.
"Importantly," the researchers say, "it also provides a proof-of-concept of how telemonitoring could be applied to monitoring respiratory diseases in general."
- Ting Dang, Jing Han, Tong Xia, Dimitris Spathis, Erika Bondareva, Chloë Siegele-Brown, Jagmohan Chauhan, Andreas Grammenos, Apinan Hasthanasombat, Andres Floto, Pietro Cicuta and Cecilia Mascolo. Exploring Longitudinal Cough, Breath, and Voice Data for COVID-19 Disease Progression Prediction via Sequential Deep Learning: Model Development and Validation. Journal of Medical Internet research (2022).