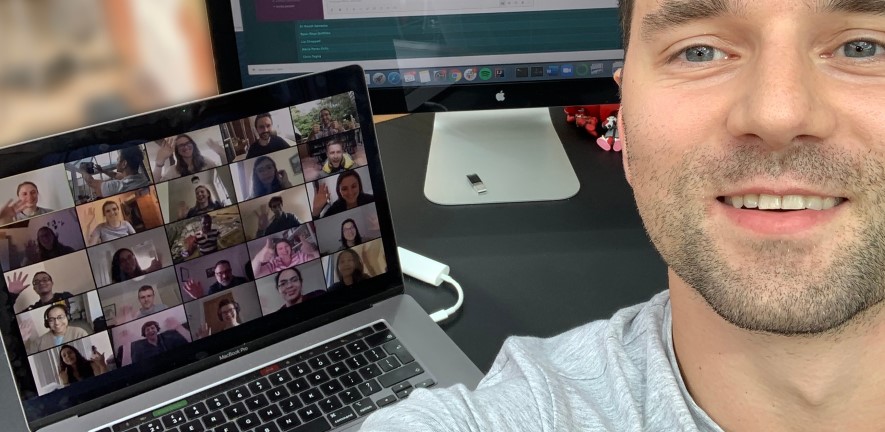
Submitted by Rachel Gardner on Wed, 14/04/2021 - 17:39
PhD students and postdocs across Cambridge have another chance to apply for a funded training course that is successfully equipping scientists with powerful tools to progress their research.
The next Data for Science Residency will run for five weeks from Monday 19 July 2021. Applications are now open and close at 9:00 am on 21 May.
The course aims to give researchers in fields outside computer science the skills they need to use machine learning (ML) in their research and helps them apply data analysis to their own datasets and problems.
The first two courses have attracted participants from fields including chemistry, biochemistry, physics, engineering, medicine, veterinary medicine and psychology.
Sheila Bhatt, a PhD student in the Department of Chemical Engineering and Biotechnology, took the most recent Data Science for Science course, which ran during February and March.
Using machine learning to develop low-cost anaemia diagnostics
She says that taking the course, and learning about the potential of applying ML techniques to the analysis of diagnostic crack-patterns in images of drying blood, has sparked the interest of her supervisor and colleagues and sparked a further international collaboration on blood diagnostics.
"The feeling is that a machine learning approach in this could be an excellent way to develop low cost, game-changing blood diagnostics, particularly for developing countries," she says.
The Data Science for Science training course is part of the new Accelerate Programme for Scientific Discovery. This is being funded by a generous donation to the University from Schmidt Futures. The Programme – which is based in the Department of Computer Science and Technology – is led by Professor Neil Lawrence, DeepMind Professor of Machine Learning.
"Machine learning and AI are increasingly part of our day-to-day lives, but they aren’t being used as effectively as they could be, due in part to major gaps of understanding between different research disciplines," Neil explains. "This Programme will help us to close these gaps by training physicists, biologists, chemists and other scientists in the latest machine learning techniques, giving them the skills they need while accelerating the excellent research already taking place here at the University."
Sheila recently began studying for a PhD with Prof Alex Routh. His group is working to understand the dynamics of drying complex colloids, like blood, and the mechanisms that govern crack-formation in drying blood droplets. (Colloids are mixtures where minute particles of one substance are dispersed throughout a second substance.)
"The feeling is that a Machine Learning approach in this could be an excellent way to develop low-cost, game-changing blood diagnostics - particularly for developing economies"
PhD student Sheila Bhatt
"Investigating the potential of analysing crack-pattern images for diagnosis of a range of disorders (both human and animal) leads to large volumes of data," Sheila says.
"If this can be analysed to reliably identify disease-states, then the potential of developing low-cost diagnostics for many disorders will be enormous – particularly in the developing world. The group's work in this area on low-cost anaemia diagnostics for developing countries has very recently attracted the interest of the Centre for Global Equality."
But crack-patterns are extremely complex and sensitive to blood-properties, "which makes the problem very challenging," she says. So she applied to take part in the Data Science for Science course, feeling that image-recognition and machine learning might have an important role to play in crack-pattern analysis.
She found the course extremely useful. "My prior computer experience was severely outdated, and the programme gave me the opportunity to update old skills and learn completely new ones."
And as a result of it, her supervisor Prof Routh – whose group already collaborates with Monash University, Australia, and with the Veterinary School and the Cavendish Laboratory here in Cambridge – has instigated a new collaboration with the University of Rome, specifically to work on the image-analysis aspects.
Interacting with other PhD students
Sheila adds that doing the course while still in the very early stages of her PhD programme had additional benefits. "Because I am just starting, I don't yet have a great deal of data. But I found it highly useful to attend at this early stage, as it allowed me to evaluate the performance of the algorithms on preliminary images and think about the data and metadata that could ensure that future image-data collection would meet the standards required for ML analysis," she says.
"It was also very useful to interact with other PhD students in the Residency as most were further along in their research and asked insightful questions."
Data for Science course participants should be current PhD students or researchers at the University of Cambridge and should have basic programming skills. Applicants are asked to commit to dedicating at least 30 hours per week to the course over the five weeks from Monday 19th July. Places are limited and participants will be selected based on a technical assessment and alignment with the aims of the programme.
To apply, candidates should complete this form by 9:00 am on 21 May. For further information, please see the FAQs.