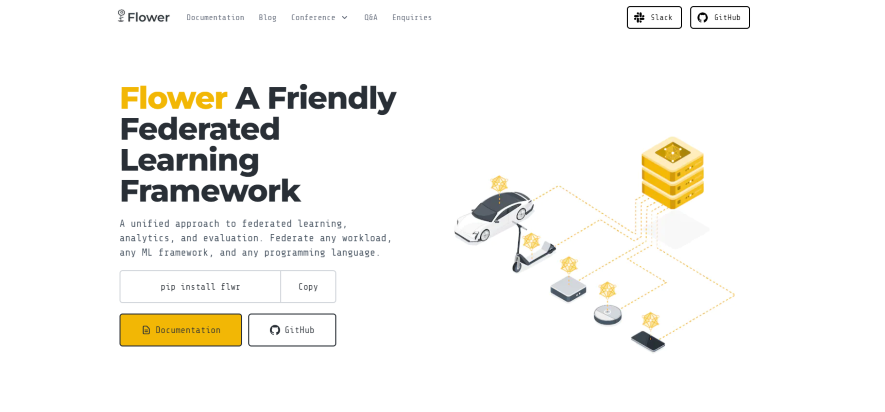
Submitted by Rachel Gardner on Tue, 20/09/2022 - 13:04
Researchers here are seeking up to 500 volunteers for an experiment that could help shape the future of private digital advertising. Could you help them out by taking part?
The study is being led by researchers in the Cambridge Machine Learning Systems Lab here. They are collaborating with partners scientists and engineers at Brave, an open-source privacy-focused browser, and Flower, an open source ‘federated learning’ framework.
Federated Learning is a technique for training machine learning models across devices without sharing the raw data they hold. With concerns about data sharing and data privacy growing over the last few years, there has been increasing interest in it and it was recently added to the MPhil in Advanced Computer Science course here.
However, federated learning is not yet in mainstream use within online advertising – which is often driven by personal user information. This study seeks to understand its potential effectiveness and system design considerations through an opt-in experiment utilising the privacy-focused browser Brave, which has over 50 million users worldwide.
Brave already has a privacy-preserving advertisement serving system, called Brave Ads, which serves ads as push notifications – but this system does not currently leverage user information within operation of the advertising system.
One of the core innovations underpinning the experiment is the efficient integration of Flower inside the Brave browser.
This effort was driven by MPhil student Andreea Zaharia (pictured left) during the past year – for which work she received the Examiners' Prize for the Best Part III project in the Department.
After considerable work in testing the system, the study is ready to be used by real world Brave users during their daily web browsing activities. The study is looking for participants to try it out and see if federated learning could be an effective way to determine when adverts should be released to the users.
"This is done by training a global ad timing model without ever having the users' data leave their devices," says Senior Research Associate on the project, Pedro Porto Buarque de Gusmao (pictured right). "With participants' help, we can prove this works and lead the way towards more private digital advertising."
This is a pilot study. If successful, "the next step would be to scale it up to a more realistic, complete federated system," says Professor Nic Lane, who runs the Cambridge Machine Learning Systems Lab here.
"The integration of the Flower client, and the scalability of the Flower aggregation server, mean that it would be relatively easy to run an experiment with five million users in the next round if this first experiment is successful. All of the technical pieces are now in place, this is tremendously exciting!"
The recruitment of volunteers to take part in the study is now underway. The researchers are seeking 500 participants to take part in the 28-day experiment. Participants must be at least 18 years old and must live in one of the Brave Ads Supported Countries. They need either to be a user of Brave and/or Brave Ads, or willing to install it.
If they opt in to take part in the experiment, low-sensitivity data will be collected locally on their browser. This will be data such as the number of tabs they have open (but not what's in them), what time of the day they clicked on an ad (but not what ad), and how long they waited before dismissing an ad.
No data about users' browsing history will be recorded for this user study. And no personally identifiable information will be collected other than participants' email address – which will be used only to direct them to the post-user study questionnaire.
After 28 days, volunteers will have the option to share with the research team the data that has been collected on their devices. They will also be asked to fill in a short post-user study questionnaire.
- If you’re interested in taking part in this experiment, you can find out more at https://mlsys.cst.cam.ac.uk/cl-brave-flower