Understanding 'fairness' in machine learning algorithms
Featured researcher: Aviva PhD student Michelle Seng Ah Lee
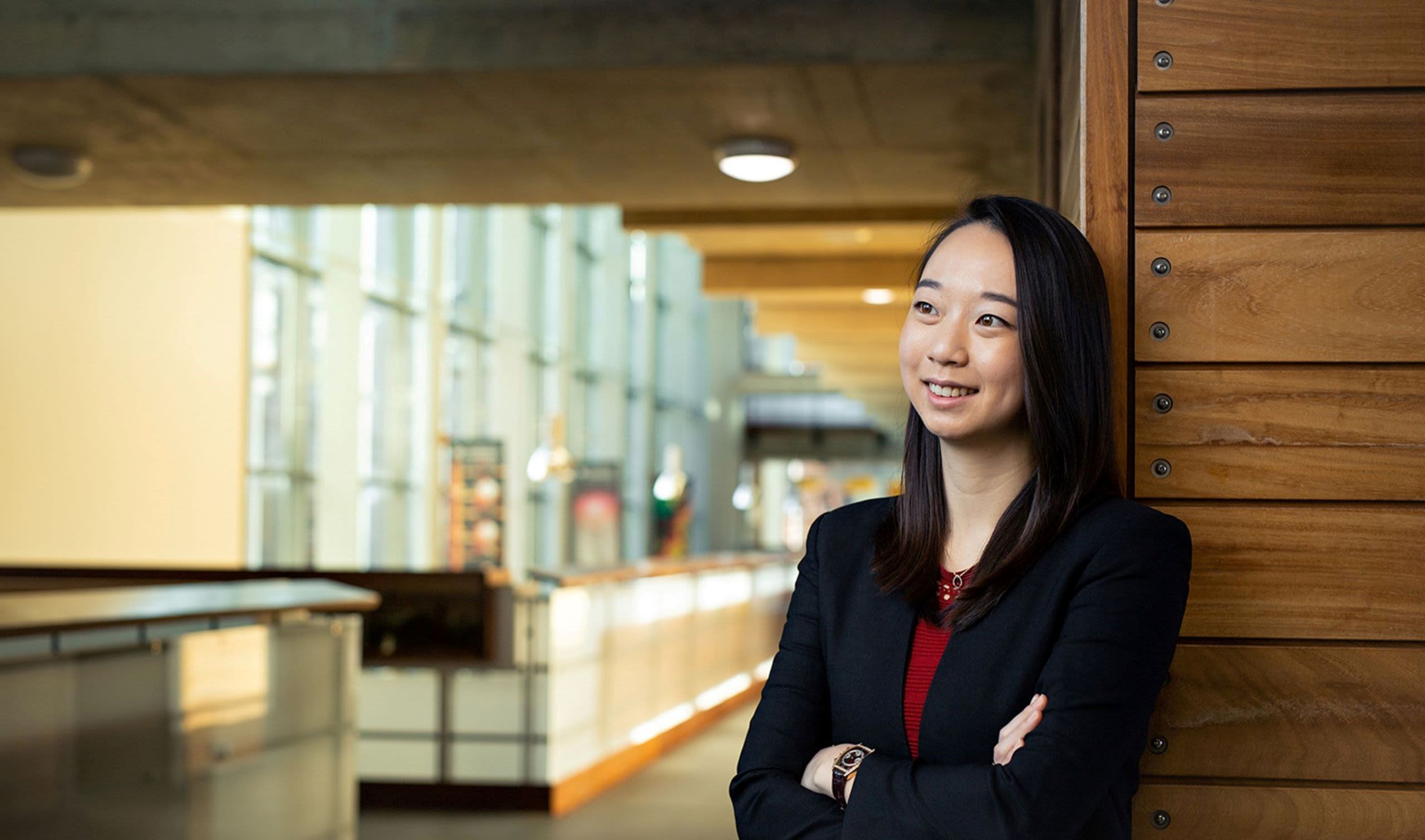
Michelle Seng Ah Lee is trying to understand fairness and how the concept can be reflected in the algorithms used by businesses.
A PhD student here (in the Compliant and Accountable Systems group, supervised by Dr Jat Singh and Professor Jon Crowcroft), she is also one of the first PhDs in Cambridge to be sponsored by the insurance firm Aviva, which recently established a partnership with the University.
Her PhD research focuses on fairness in machine learning algorithms and their trade-offs on aggregate and individual levels.
Though you might not think of insurance companies as being at the cutting edge of data science, in fact such businesses have been running on big data and algorithms for years. And as data science continues to advance, Aviva wants to remain at its forefront, both to improve the way it does things in the short term but also to look afresh at the whole business of insurance and its role in society.
As part of the partnership with Cambridge, Aviva is sponsoring three PhD students including Michelle who is researching ways that the algorithms used by firms like Aviva can remain fair.
Michelle herself has a foot in industry as AI Ethics Lead at a 'Big Four' professional services firm. She is also actively involved in DataKind, a global charity that seeks to harness advances in data science for societal benefit. Michelle is interested in helping firms design algorithms that reflect their values.

Fairness seems like an important notion as more and more decisions – from recommending new products to approving a loan to hiring a new employee – are informed by machines and models.
"There are some topics – such as data ethics – where it is really helpful having Cambridge input. This is such an important subject we need to have not just our own view but also an impartial, world-class academic view."
Dr Orlando Machado, Chief Data Scientist, Aviva PLC
While these models can tackle discrimination and subconscious human bias in processes, Lee contends that ‘fairness’ is a challenging concept to define in an algorithm. One person’s idea of fairness is not necessarily the same as everyone else’s: value judgements are involved. She cites a well-known example of two competing views of fairness in the US Criminal Justice System.
"An algorithm widely used to forecast future criminal behavior was under scrutiny because black offenders were found to be twice as likely to be incorrectly labelled as having a higher risk of repeat offending than white defendants.
"However, the company that created the algorithm maintains that it is non-discriminatory because the rate of accuracy for its scores is identical for black and white defendants.
"While both perspectives sound fair they are based on different perceptions of what fairness means, and it is mathematically impossible to meet both objectives at the same time."
And that’s what Lee’s work is trying to do: by acknowledging that fairness is not a universal concept, she wants to make transparent the values, risk and impact underpinning each algorithm so that managers can decide what kind of trade-offs they want to make and what controls and governance need to be put in place.
“If, for example, you are building an insurance pricing algorithm, there needs to be a way of saying to senior executives: ‘These are your options. If you choose this one, you will lower your portfolio risk and make insurance cheaper for your customers overall but it may have consequences for these groups. Here's how we address this risk.’
“My goal is to come up with a practical way for people to assess whether an algorithm supports their firm’s values. My code and methodologies will be made available as open source for anyone developing decision-making processes or algorithms.”
- This story is adapted from the article ‘Strategic Partner: Aviva / Insuring Its Future’ on the University of Cambridge School of Technology website.
- Photos: StillVision Photography