Amanda Prorok, robot trainer
Meet this month's featured researcher
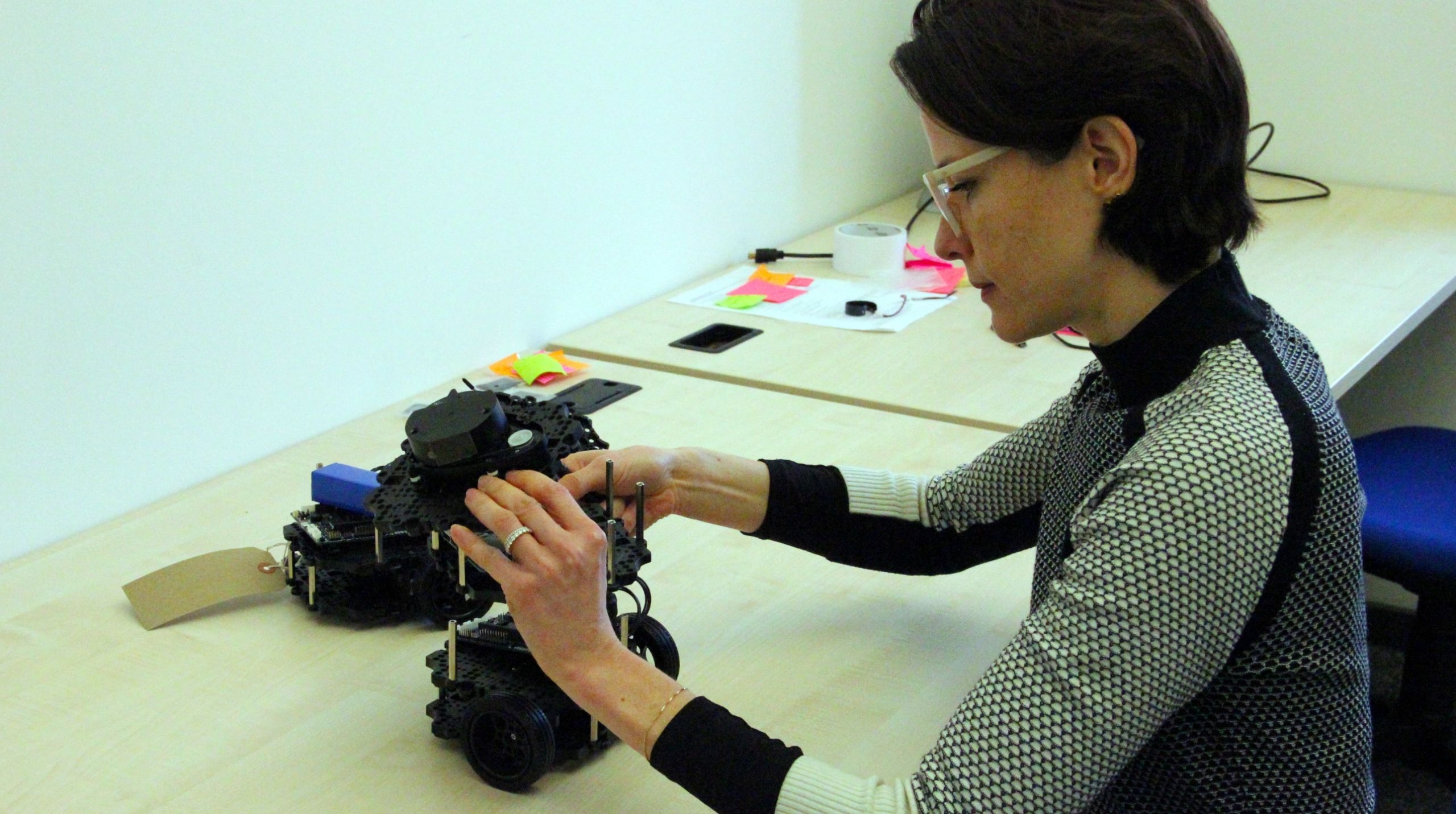
Encountering the concept of ‘swarm intelligence’ at university was a life-changing moment for Dr Amanda Prorok. “It really resonated with me,” she says. “I knew if there was one idea I’d want to work on for the rest of my life, this was it.”
Today an Assistant Professor and University Lecturer in Cyber-Physical Systems here, Amanda leads award-winning research into multi-agent and multi-robot systems. Her group looks for new ways to induce artificially intelligent agents – such as robots, machines and driverless cars – to achieve common goals while working in shared spaces like warehouses and roads. She has just won a 2019 Amazon Research Award to try and solve a currently intractable problem in this area. Here she talks about her work.
My interest in robotics and collective intelligence stems from my postgraduate studies. After completing my first degree in Zurich (where I'd grown up, the child of a British father and a Brazilian mother), I went on to EPFL, the Swiss federal institute of technology in Lausanne. Professor Alcherio Martinoli taught a class there called ‘Swarm Intelligence’ – and the concept completely resonated with me. I started studying with him and loved it, and was very happy when he offered me a PhD there.
I studied multi-robot systems, trying to solve the positioning problem of having a group of mobile robots find out where they are in a space when they all have different capabilities. I wanted to understand how one could exploit the complementarities between diverse robots with different sensing capabilities. [Her research, on localization with ultra-wideband sensing for robotic networks, won the 2014 ABB award for the best thesis in Computer Science, Automatics, and Telecommunications.]
My subsequent three years at the University of Pennsylvania, where I went after EPFL, were really formative and influential for my career. I was a postdoctoral researcher, working with Professor Vijay Kumar (a luminary in the field of robotics) on developing a deeper understanding of the relationship between heterogeneity, diversity, and resilience in multi-robot systems.
Dr Amanda Prorok giving a talk on Diversity and Resilience in Robot Networks at the University of California, San Diego, in 2017.
Multi-robot systems are basically just one physical manifestation of collective intelligence. In my group, we’re interested in how we get different autonomous agents to work with each other to solve problems. When each has their own policies or algorithms that decide their behaviour, we look at what conditions we need to create to get these agents to work together to achieve desirable goals.
Some of our work in this area went viral last year. I and two undergraduates, Nicholas Hyldmar and Michael He, programmed a small fleet of miniature robotic cars to drive on a two-lane track and observed how the traffic flow changed when one of the cars stopped.
When the cars were not driving cooperatively, queues quickly formed behind the stationary car. But when they were driving cooperatively and communicating with each other, it was a different story. When one car stopped in the inner lane, it sent a signal to the other cars. Those driving in the outer lane slowed slightly, allowing cars in the inner lane to pull out past the stationary vehicle without having to queue. Overall, we found that a fleet of driverless cars working together like this to keep traffic moving smoothly can improve overall traffic flow by at least 35 per cent.
But this is just one facet of a very broad concept. Collective intelligence branches out into policy-making (another area of interest to me, which is why I am also part of the Cambridge Centre for Science and Policy). It branches out into mixed human-agent systems – such as the ones we are going to see in the next couple of decades when we will have a mixture of both human-driven and driverless cars on the roads.
Cars were one of my first avenues of pursuit because it is one of the robotics/automation technologies that is closest to being realised in the real world. With our research, we developed fundamental algorithms that led to us demonstrating something of real value to society – namely, that autonomous agents can work together to achieve higher-order goals such as efficient and collision-free navigation.
We are continuing to work in this area, looking at what will happen when all driverless cars are heterogeneous – a very likely scenario when each automotive manufacturer will have their own vision of how they want to implement autonomy in their driverless cars. (See her latest research - on 'Effects of Controller Heterogeneity on Autonomous Vehicle Traffic' - here.)
We are also branching out into an area that’s a bit further out: logistics. And we’re thinking not just about robotic autonomous agents in warehouses, but on a much broader scale, where the whole chain is automated and mobilised through autonomous agents. I’m very interested in understanding how we would need to coordinate those autonomous agents to make logistics more efficient.
I have just won a 2020 Amazon Research Award to progress a project on ‘Learning Explicit Communication for Multi-Robot Path Planning’. This is about coordinating robots in a cluttered space – like a warehouse – using machine learning. We are trying to understand how we can get robots to make partial plans locally and share them with others in their vicinity. The aim is that within their local environment they can get out of each other’s way and still achieve the goals in disruption-free, collision-free efficient manners.
From the planning perspective, it’s exceptionally complex to coordinate a multi-robot system in which different robots go to diverse locations in a physical workspace, collect something and return with it, while also negotiating fixed obstacles (such as shelving) and dynamic moving obstacles (such as humans).
This problem belongs to the set of problems mathematically described as NP_Hard: essentially, as you scale up this system, it becomes intractable. Currently, you cannot hope to solve these problems in real time with any more than a few tens of agents.
My research is about trying to promote efficiency and optimal performance through using data-driven ways of tackling the problem. So what I propose is to both solve the intractability of the problem – by employing a machine-learning method to get around this, instead of classical search-based algorithms – but also exploiting local computation in order to help out with the whole computational procedure. This builds on earlier work I have done using graph neural networks for decentralized multi-robot path planning.
Back to all news.